How Predictive Analytics Improves Financial Forecasting
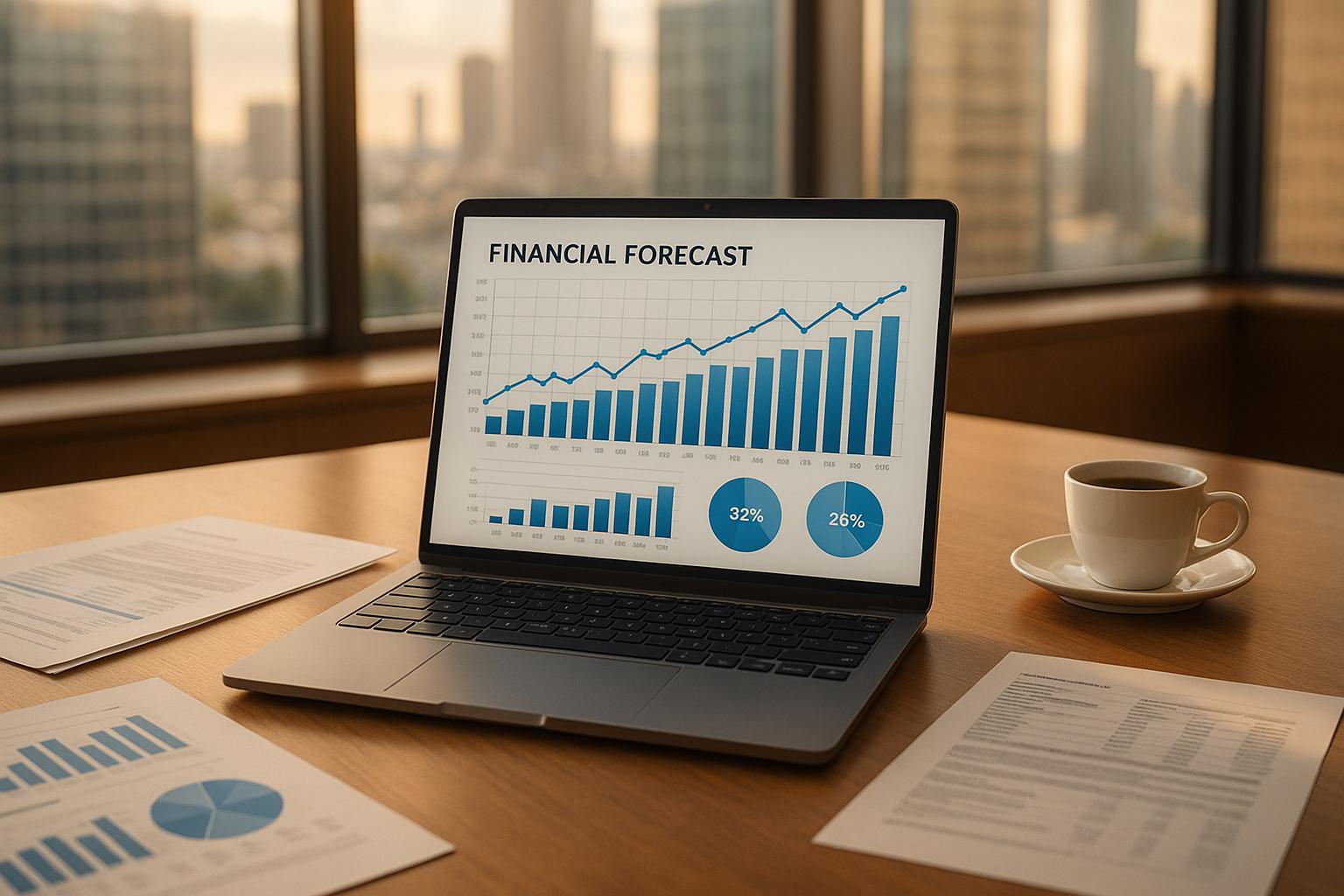
Predictive analytics helps businesses make better financial decisions by using AI and machine learning to analyze historical and real-time data. It improves accuracy, detects risks early, and supports smarter planning for growth-stage companies. Key benefits include:
- Accurate Forecasts: Identifies patterns to set realistic, data-backed goals.
- Better Decisions: Allocates resources effectively and builds reliable financial systems.
- Early Risk Detection: Spots cash flow issues, budget overruns, and market changes early.
- Integrated Insights: Combines internal metrics with macroeconomic data for a complete financial view.
To get started, unify your data, choose the right prediction models (like time series or machine learning), and regularly refine your forecasts. This structured approach turns raw data into actionable insights, helping companies grow and stay ahead of risks.
Benefits of Predictive Analytics in Forecasting
Predictive analytics has made a strong impact on FP&A (Financial Planning and Analysis), offering clear advantages when it comes to forecasting.
More Accurate Forecasts
By analyzing historical data, predictive analytics identifies complex business patterns, making forecasts more actionable and grounded in reality. It also helps set realistic, data-backed KPIs.
"Hire PSG if you want to make your life easier and have accurate data." - Michael Mancuso, CIO, New Law Business Model
Smarter Business Decisions
With insights drawn from data, executives can fine-tune strategies and allocate resources more effectively. Incorporating predictive analytics into FP&A allows businesses to:
- Build financial systems that maintain themselves
- Design revenue models based on proven data
- Fine-tune performance metrics for teams
- Strengthen their position for higher valuations
Turning raw data into meaningful business insights helps organizations track financial health and spot performance trends. These insights enable better decision-making and allow companies to address potential risks before they escalate.
Early Risk Detection
One of the standout features of predictive analytics is its ability to identify risks early. Through ongoing data analysis, management can be alerted to:
- Unusual cash flow trends
- Budget overruns
- Revenue gaps
- Shifting market conditions
"If you want to sleep better at night, hire Phoenix Strategy Group." - Patrick Wallain, Founder / CEO, ABLEMKR
Implementing Predictive Analytics
To successfully use predictive analytics for financial forecasting, you need a structured approach that focuses on managing data, selecting the right models, and constantly refining your process. This approach transforms insights into practical actions.
Data Sources and Collection
Set up systems to gather all necessary data, including:
- Financial metrics like revenue, expenses, and cash flow
- Sales and marketing performance data
- Customer and operational metrics
- Market indicators
Automate and standardize data collection to ensure consistency across departments. This avoids fragmented information that could lead to incomplete or inaccurate forecasts.
Choosing Prediction Models
Select prediction models based on your business goals and the quality of your data. Start simple and increase complexity as needed:
Model Type | Best For | Key Requirements |
---|---|---|
Time Series Analysis | Identifying regular patterns | Reliable historical data |
Regression Models | Forecasting revenue | Clearly defined variable relationships |
Machine Learning | Detecting complex patterns | Large, high-quality datasets |
Improving Model Accuracy
Boosting model accuracy requires ongoing monitoring and updates. A weekly review cycle is a good starting point to compare forecasts with actual results:
1. Data Quality Assessment
Regularly audit your data sources to ensure inputs are complete and reliable.
2. Performance Metrics Tracking
Keep an eye on key performance indicators (KPIs) and adjust forecasts accordingly.
3. Continuous Refinement
Adapt your models based on new data and evolving business conditions. Regular updates help keep your forecasts accurate and aligned with current realities.
The effectiveness of predictive analytics hinges on team collaboration and consistent execution. By sticking to these structured methods and maintaining frequent reviews, businesses can achieve more accurate forecasts and make smarter decisions.
sbb-itb-e766981
Using Macroeconomic Data in Forecasts
Key Economic Indicators
Incorporating macroeconomic data into predictive models adds essential context for more precise financial forecasting. Some of the most impactful economic indicators include:
Economic Indicator | Impact on Forecasting | Update Frequency |
---|---|---|
GDP Growth Rate | Tracks market expansion/contraction | Quarterly |
Interest Rates | Influences borrowing costs | Monthly |
Industry-Specific Indices | Highlights sector trends | Monthly |
Consumer Price Index | Guides pricing strategies | Monthly |
Employment Data | Reflects consumer spending power | Monthly |
These indicators provide external context that complements your internal data. When combined, they enhance the accuracy of financial projections.
Merging Internal and External Data
Blending external economic indicators with your internal metrics offers a more comprehensive view of potential opportunities and risks. Predictive analytics becomes far more effective when these data sets are integrated.
Here’s how to make this integration work:
-
Streamline Data Collection
Build a unified dashboard that combines internal and external metrics. This ensures everyone in your organization works with consistent, accurate information. -
Define Key Metrics
Identify the most relevant combinations of internal and external data for your business. Regularly review these to ensure alignment across teams.
"If you want to sleep better at night, hire Phoenix Strategy Group." – Patrick Wallain, Founder/CEO of ABLEMKR
- Use Adaptive Models
Develop financial models that adjust dynamically as new data becomes available. This flexibility helps maintain forecast accuracy even during market fluctuations.
To succeed, focus on consistent data quality and regular updates. Automating and standardizing data collection, setting up review cycles, and creating scalable systems for new data sources are critical steps. This approach turns raw data into actionable insights, enabling smarter decisions and more accurate growth forecasts.
Conclusion
Key Takeaways
Predictive analytics has transformed financial forecasting for growth-stage companies, offering the ability to make precise, data-driven decisions. By blending internal metrics with macroeconomic data, businesses can:
- Develop accurate growth projections
- Spot risks early
- Align teams with actionable goals
- Make decisions rooted in solid data
The combination of historical data with forward-looking indicators creates a flexible forecasting system that adjusts to market shifts, providing a strong foundation for decision-making.
How to Get Started
To start integrating predictive analytics into your financial processes, follow these steps:
1. Unify Your Data
Bring together financial, sales, marketing, and operational data in one system to ensure reliable inputs for your predictive models.
2. Build Analytics Systems
Implement systems to continuously collect and process data, focusing on metrics that directly support your business goals.
3. Turn Insights Into Action
Use your forecasts to drive results by focusing on these areas:
Focus Area | Actions | Outcome |
---|---|---|
Data Quality | Regular audits and updates | Accurate and dependable insights |
Team Alignment | Weekly tracking, monthly planning | Consistent execution |
Model Refinement | Ongoing testing and adjustments | Better forecast reliability |
"Hire PSG if you want to make your life easier and have accurate data." - Michael Mancuso, CIO, New Law Business Model
Phoenix Strategy Group offers fractional CFO services, combining expertise and advanced tools to help growth-stage companies scale effectively.
The journey to better financial forecasting starts with the right data and a capable team. By following these steps and seeking expert support, businesses can build a reliable predictive analytics framework to fuel growth and drive success.
FAQs
How can businesses improve the accuracy of predictive analytics in financial forecasting?
To improve the accuracy of predictive analytics in financial forecasting, businesses should focus on three key areas:
- Data Quality: Ensure that the data used is accurate, up-to-date, and relevant. This includes incorporating both internal financial data and external macroeconomic indicators.
- Model Validation: Regularly test and validate predictive models using historical data to ensure they perform as expected. Adjust models as needed to account for changing market conditions or new data trends.
- Expert Oversight: Combine machine learning insights with expert analysis. Human expertise is essential for interpreting results and identifying potential anomalies or biases in the data.
By leveraging these practices, businesses can make more reliable financial forecasts, enabling better decision-making and strategic planning.
What’s the difference between time series analysis, regression models, and machine learning in financial forecasting?
Time series analysis, regression models, and machine learning are all powerful tools for financial forecasting, but they serve different purposes and work best in specific scenarios.
- Time series analysis focuses on historical data trends over time, making it ideal for identifying seasonality and long-term patterns. It assumes that past behavior is a good predictor of future outcomes.
- Regression models examine relationships between variables, helping to understand how factors like macroeconomic indicators impact financial performance. These models are great for testing hypotheses and making projections based on known relationships.
- Machine learning leverages algorithms to analyze large datasets, uncover hidden patterns, and make predictions. Unlike traditional methods, it adapts and improves over time, offering more accurate and dynamic forecasting, especially when dealing with complex or non-linear data.
By combining these approaches, businesses can create more robust and reliable financial forecasts tailored to their unique needs.
How does combining macroeconomic data with internal metrics improve financial forecasting?
Combining macroeconomic data with internal metrics improves financial forecasting by offering a more comprehensive view of both internal performance and external market conditions. By factoring in trends like inflation, interest rates, and GDP growth, businesses can better anticipate how economic shifts might impact their operations.
This approach enables more accurate growth projections and helps organizations make informed decisions about budgeting, investments, and long-term strategy. It ensures forecasts are not just based on past performance but also aligned with the broader economic environment.